University of Florence
Course on Multimedia Databases – 2013/14 (Prof. A. Del Bimbo)
Instructors: Lamberto Ballan and Lorenzo Seidenari
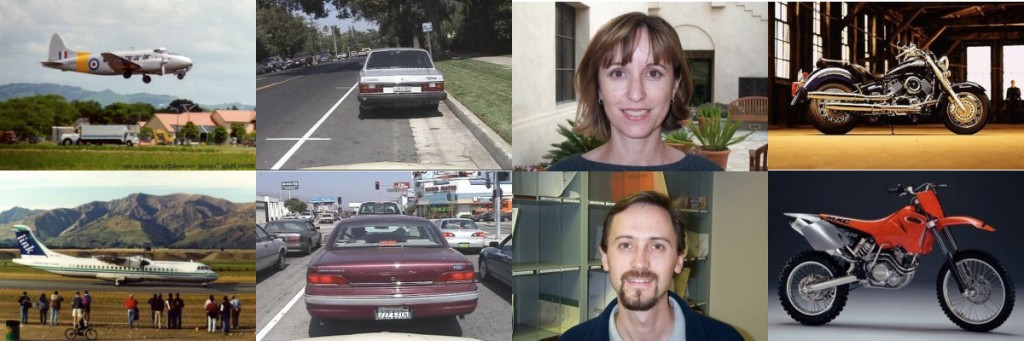
Goal
The goal of this laboratory is to get basic practical experience with image classification. We will implement a system based on bag-of-visual-words image representation and will apply it to the classification of four image classes: airplanes, cars, faces, and motorbikes.
We will follow the three steps:
- Load pre-computed image features, construct visual dictionary, quantize features
- Represent images by histograms of quantized features
- Classify images with Nearest Neighbor / SVM classifiers
Getting started
- Download excercises-description.pdf
- Download lab-bow.zip (type the password given in class to uncompress the file) including the Matlab code
- Download 4_ObjectCategories.zip including images and precomputed SIFT features; uncompress this file in lab-bow/img
- Download 15_ObjectCategories.zip including images and precomputed SIFT features; uncompress this file in lab-bow/img
- Start Matlab in the directory lab-bow/matlab and run exercises.m
Our paper entitled “Context-Dependent Logo Matching and Recognition” – by H. Sahbi, L. Ballan, G. Serra and A. Del Bimbo – has been accepted for publication in the IEEE Transactions on Image Processing (pdf, link). Part of this work was conducted while me and G. Serra were visiting scholars at Telecom ParisTech (in spring 2010).
We contribute through this paper to the design of a novel variational framework able to match and recognize multiple instances of multiple reference logos in image archives. Reference logos as well as test images, are seen as constellations of local features (interest points, regions, etc.) and matched by minimizing an energy function mixing (i) a fidelity term that measures the quality of feature matching (ii) a neighborhood criterion which captures feature co-occurrence/geometry and (iii) a regularization term that controls the smoothness of the matching solution. We also introduce a detection/recognition procedure and we study its theoretical consistency. We show the validity of our method through extensive experiments on the novel challenging MICC-Logos dataset overtaking, by 20%, baseline as well as state-of-the-art matching/recognition procedures. We present also results on another public dataset, the FlickrLogos-27 image collection, to demonstrate the generality of our method.
Our paper “Combining Generative and Discriminative Models for Classifying Social Images from 101 Object Categories” has been accepted at ICPR’12. We use a hybrid generative-discriminative approach (LDA + SVM with non-linear kernels) over several visual descriptors (SIFT, GIST, colorSIFT).
A major contribution of our work is also the introduction of a novel dataset, called MICC-Flickr101, based on the popular Caltech 101 and collected from Flickr. We demonstrate the effectiveness and efficiency of our method testing it on both datasets, and we evaluate the impact of combining image features and tags for object recognition.
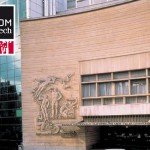
From April 6 to June 30, 2010, I will be a visiting PhD student at Telecom Paristech in Paris (France). Telecom Paristech (also known as ENST) is one of the most prestigious and selective grandes écoles in France and one of the finest institutions in the field of Telecommunications.
Together with Giuseppe Serra, we will work in the Image Processing and Interpretation (TII) group in the department of Signal and Image Processing (TSI), collaborating with Dr. Hichem Sahbi.
Our paper on “Video Event Classification using String Kernels“ was accepted for publication by Springer International Journal on Multimedia Tools and Applications (MTAP) in the special issue on Content-Based Multimedia Indexing.
In this paper we present a method to introduce temporal information for video event recognition within the bag-of-words (BoW) approach. Events are modeled as a sequence composed of histograms of visual features, computed from each frame using the traditional BoW. The sequences are treated as strings phrases where each histogram is considered as a character. Event classification of these sequences of variable length, depending on the duration of the video clips, are performed using SVM classifiers with a string kernel that uses the Needlemann-Wunsch edit distance.