Our paper “Combining Generative and Discriminative Models for Classifying Social Images from 101 Object Categories” has been accepted at ICPR’12. We use a hybrid generative-discriminative approach (LDA + SVM with non-linear kernels) over several visual descriptors (SIFT, GIST, colorSIFT).
A major contribution of our work is also the introduction of a novel dataset, called MICC-Flickr101, based on the popular Caltech 101 and collected from Flickr. We demonstrate the effectiveness and efficiency of our method testing it on both datasets, and we evaluate the impact of combining image features and tags for object recognition.
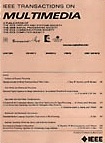
Our paper entitled “Effective Codebooks for Human Action Representation and Classification in Unconstrained Videos” by L. Ballan, M. Bertini, A. Del Bimbo, L. Seidenari and G. Serra has been accepted for publication in the IEEE Transactions on Multimedia.
Recognition and classification of human actions for annotation of unconstrained video sequences has proven to be challenging because of the variations in the environment, appearance of actors, modalities in which the same action is performed by different persons, speed and duration and points of view from which the event is observed. This variability reflects in the difficulty of defining effective descriptors and deriving appropriate and effective codebooks for action categorization.
In this paper we propose a novel and effective solution to classify human actions in unconstrained videos. It improves on previous contributions through the definition of a novel local descriptor that uses image gradient and optic flow to respectively model the appearance and motion of human actions at interest point regions. In the formation of the codebook we employ radius-based clustering with soft assignment in order to create a rich vocabulary that may account for the high variability of human actions. We show that our solution scores very good performance with no need of parameter tuning. We also show that a strong reduction of computation time can be obtained by applying codebook size reduction with Deep Belief Networks with little loss of accuracy.
Our method has obtained very competitive performance on several popular action-recognition datasets such as KTH (accuracy = 92.7%), Weizmann (accuracy = 95.4%) and Hollywood-2 (mAP = 0.451).
Our paper “Commercials and Trademarks Recognition” has been accepted as book chapter in TV Content Analysis: Techniques and Applications that will be published by CRC Press, Taylor & Francis group, on March 2012.
Book summary: TV content is currently available through various communication channels and devices, including digital TV, mobile TV, and Internet TV. However, with the increase in TV content volume, both its management and consumption become more and more challenging. Thoroughly describing TV program analysis techniques, this book explores the systems, architectures, algorithms, applications, research results, new approaches, and open issues. Leading experts address a wide variety of related subject areas and present a scientifically sound treatment of state-of-the-art techniques for readers interested or involved in TV program analysis.
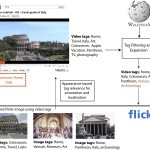
Our paper entitled “Enriching and Localizing Semantic Tags in Internet Videos” has been accepted by ACM Multimedia 2011.
Tagging of multimedia content is becoming more and more widespread as web 2.0 sites, like Flickr and Facebook for images, YouTube and Vimeo for videos, have popularized tagging functionalities among their users. These user-generated tags are used to retrieve multimedia content, and to ease browsing and exploration of media collections, e.g. using tag clouds. However, not all media are equally tagged by users: using the current browsers is easy to tag a single photo, and even tagging a part of a photo, like a face, has become common in sites like Flickr and Facebook; on the other hand tagging a video sequence is more complicated and time consuming, so that users just tend to tag the overall content of a video.
In this paper we present a system for automatic video annotation that increases the number of tags originally provided by users, and localizes them temporally, associating tags to shots. This approach exploits collective knowledge embedded in tags and Wikipedia, and visual similarity of keyframes and images uploaded to social sites like YouTube and Flickr. Our paper is now available online.
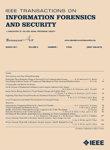
The paper “A SIFT-based forensic method for copy-move attack detection and transformation recovery” by I. Amerini, L. Ballan, R. Caldelli, A. Del Bimbo, and G. Serra is now officially accepted for publication by the IEEE Transactions on Information Forensics and Security.
One of the principal problems in image forensics is determining if a particular image is authentic or not. This can be a crucial task when images are used as basic evidence to influence judgment like, for example, in a court of law. To carry out such forensic analysis, various technological instruments have been developed in the literature.
In this paper the problem of detecting if an image has been forged is investigated; in particular, attention has been paid to the case in which an area of an image is copied and then pasted onto another zone to create a duplication or to cancel something that was awkward. Generally, to adapt the image patch to the new context a geometric transformation is needed. To detect such modifications, a novel methodology based on Scale Invariant Features Transform (SIFT) is proposed. Such a method allows both to understand if a copy-move attack has occurred and, furthermore, to recover the geometric transformation used to perform cloning. Extensive experimental results are presented to confirm that the technique is able to precisely individuate the altered area and, in addition, to estimate the geometric transformation parameters with high reliability. The method also deals with multiple cloning.
More information about this project (there are also links to datasets used in the experiments) are available on this page.
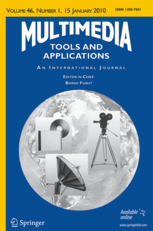
Our paper “Event detection and recognition for semantic annotation of video” was accepted for publication by Springer International Journal of Multimedia Tools and Applications (MTAP) in the special issue “Survey papers in Multimedia by World Experts”. The paper is available online now (see publications) and it is also available on SpringerLink (DOI).
In this paper we survey the field of event recognition, from interest point detectors and descriptors, to event modelling techniques and knowledge management technologies. We provide an overview of the methods, categorising them according to video production methods and video domains, and according to types of events and actions that are typical of these domains.
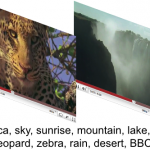
Our paper on “Tag suggestion and localization in user-generated videos based on social knowledge” won the best paper award at ACM SIGMM Workshop on Social Media (WSM’10) in conjunction with ACM Multimedia 2010.
Nowadays, almost any web site that provides means for sharing user-generated multimedia content, like Flickr, Facebook, YouTube and Vimeo, has tagging functionalities to let users annotate the material that they want to share. The tags are then used to retrieve the uploaded content, and to ease browsing and exploration of these collections, e.g. using tag clouds. However, while tagging a single image is straightforward, and sites like Flickr and Facebook allow also to tag easily portions of the uploaded photos, tagging a video sequence is more cumbersome, so that users just tend to tag the overall content of a video. While research on image tagging has received a considerable attention in the latest years, there are still very few works that address the problem of automatically assigning tags to videos, locating them temporally within the video sequence.
In this paper we present a system for video tag suggestion and temporal localization based on collective knowledge and visual similarity of frames. The algorithm suggests new tags that can be associated to a given keyframe exploiting the tags associated to videos and images uploaded to social sites like YouTube and Flickr and visual features. Our paper is now available online.
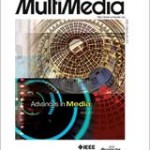
Our paper on “Video Annotation and Retrieval Using Ontologies and Rule Learning” was accepted for publication by the International IEEE MultiMedia Magazine.
In this paper we present an approach for automatic annotation and retrieval of video content, based on ontologies and semantic concept classifiers. A novel rule-based method is used to describe and recognize composite concepts and events. Our algorithm learns automatically rules expressed in Semantic Web Rules Language (SWRL), exploiting the knowledge embedded into the ontology. Concepts’ relationship of co-occurrence and the temporal consistency of video data are used to improve the performance of individual concept detectors. Finally, we present a web video search engine, based on ontologies, that permits queries using a composition of boolean and temporal relations between concepts.
Our paper on “Video Event Classification using String Kernels“ was accepted for publication by Springer International Journal on Multimedia Tools and Applications (MTAP) in the special issue on Content-Based Multimedia Indexing.
In this paper we present a method to introduce temporal information for video event recognition within the bag-of-words (BoW) approach. Events are modeled as a sequence composed of histograms of visual features, computed from each frame using the traditional BoW. The sequences are treated as strings phrases where each histogram is considered as a character. Event classification of these sequences of variable length, depending on the duration of the video clips, are performed using SVM classifiers with a string kernel that uses the Needlemann-Wunsch edit distance.
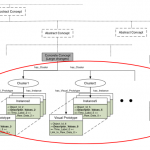
Our paper on “Semantic annotation of soccer videos by visual instance clustering and spatial/temporal reasoning in ontologies” was accepted for publication by Springer International Journal on Multimedia Tools and Applications (MTAP).
In this paper we present a framework for semantic annotation of soccer videos that exploits an ontology model referred to as Dynamic Pictorially Enriched Ontology, where the ontology, defined using OWL, includes both schema and data. Visual instances are used as matching references for the visual descriptors of the entities to be annotated. The paper is available online now and it is also available on SpringerLink in the “Online First” section (DOI).